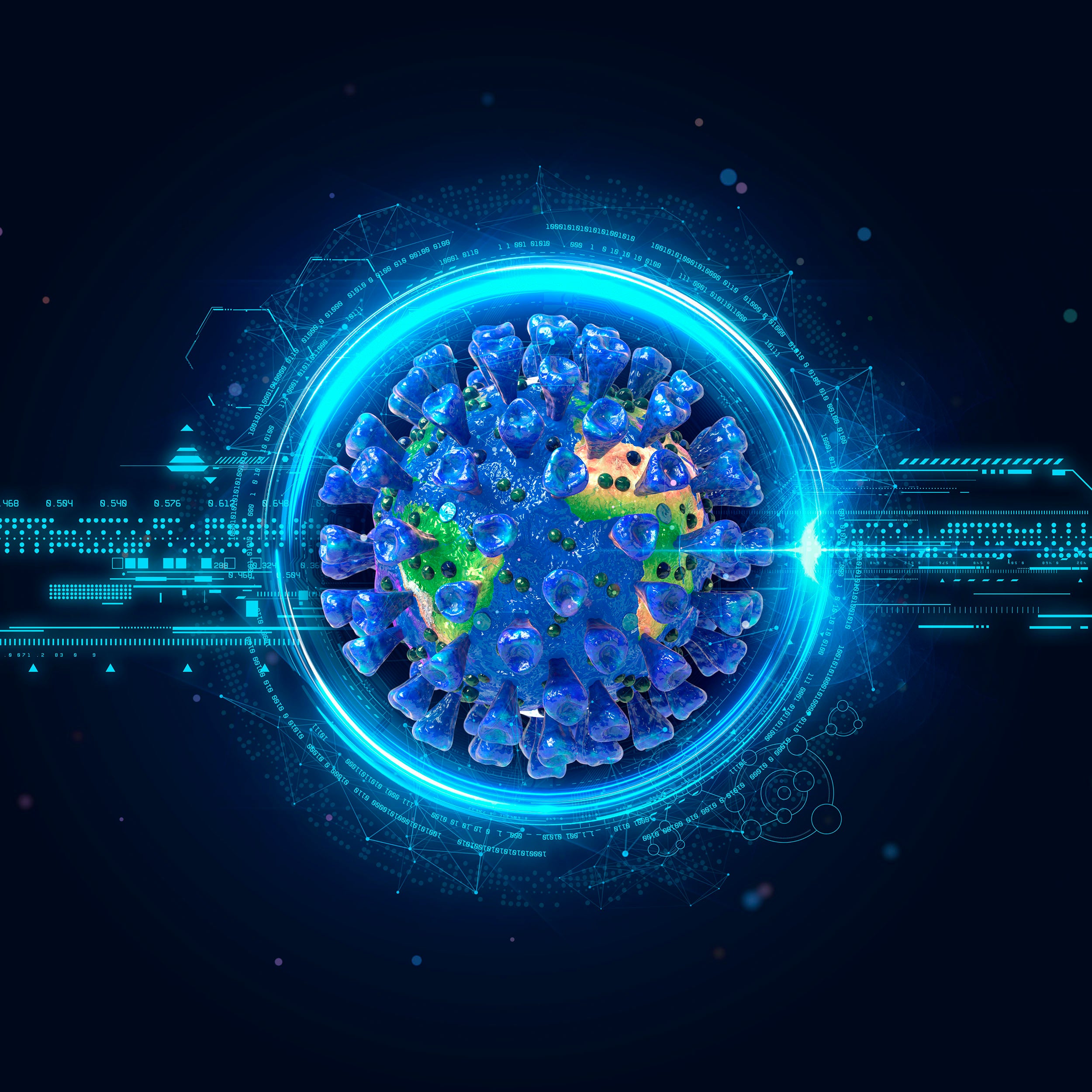
Illustration by Oliver Burston
Health
Using AI as a pandemic crystal ball
MGH, Broad researchers develop Big Data tool that can predict which variants will likely trigger surges
A team of researchers recently developed an artificial intelligence model that can predict which coronavirus variants will likely dominate and cause surges. The work was led by Jacob Lemieux, an assistant professor of medicine at Harvard Medical School and Massachusetts General Hospital, and Pardis Sabeti, a member at the Broad Institute of MIT and Harvard, professor of organismic and evolutionary biology at Harvard’s Faculty of Arts and Sciences, and of immunology and infectious diseases at the Harvard T.H. Chan School of Public Health. It also benefits from the work of AI researchers Fritz Obermeyer and Martin Jankowiak, who joined the Broad in 2020 from Uber AI Labs, where they developed a machine-learning model that can handle massive amounts of data and provided a foundation for the latest work. The Gazette spoke with Lemieux and Sabeti about the new AI/machine-learning model, called PyR0 (pie-R-naught) and how it will help in the current pandemic and for diseases to come.
Q&A
Jacob Lemieux and Pardis Sabeti
GAZETTE: You and colleagues have developed a machine-learning model that predicted the emergence of at least two particularly transmissible SARS-CoV-2 variants that caused a lot of illness globally. Can you tell us a little bit about that?
LEMIEUX: The clearest prediction that the model made was that, among the Omicron sub-lineages, BA.2 was the fittest. At the time that we analyzed the data, it was a BA.1 (Omicron) epidemic and BA.1 was the variant that everyone was focused on — in South Africa initially and then just about everywhere else in the world. The model made a fairly strong and confident prediction that BA.2 was fitter. That was based on BA.2’s dynamics in a few locations, mainly India and Denmark, which turned out to be quite accurate. Since that time, BA.2 has taken over BA.1 just about everywhere, and BA.4 or 5 are actually sub-lineages of BA.2. That was a vote of confidence in the model’s ability to at least forecast dynamics.
We also conducted an analysis — looking in retrospect — of what the model would have said is going to happen in different regions and globally. And the model would have picked up the alpha variant, B.117, and it would have picked up delta, around the same time that these lineages were picked up by leading, highly collaborative, and very labor-intensive surveillance efforts. So, we think it’s complementary to but doesn’t replace people staring really hard at the data and fitting focused models on individual regions. The nice thing is that it can compute all the data at once and aggregate information across regions, which is something that can be hard for a single person to do. It’s a useful tool in that regard.
“Whenever people ask, ‘Have we generated too much data?’, I argue that we haven’t by a long shot.”
Pardis Sabeti
GAZETTE: With BA.4 and BA.5 taking off this summer, what have recent runs told you about the course of the pandemic to come?
LEMIEUX: The model currently suggests that BA.2.75 is one to watch, although it doesn’t think the fitness differences are too great relative to other circulating variants. This suggests BA.2.75 may take over in some places but probably won’t change the pandemic in a major way.
GAZETTE: Does it say anything about disease severity?
LEMIEUX: Nothing. Growth rate is just one microbial phenotype. but there are so many other microbial phenotypes, like disease severity, that probably also have a genetic basis and that hopefully we’re going to be able to figure out using approaches like this. There’s already been a lot of work in this area for drug resistance, that’s been the one where we’ve had a good link between microbial genotype and microbial phenotype. So, I’m optimistic that with the growing scale of data and the new algorithmic tools and increasing computing power, we’ll be able to tackle some of these questions.
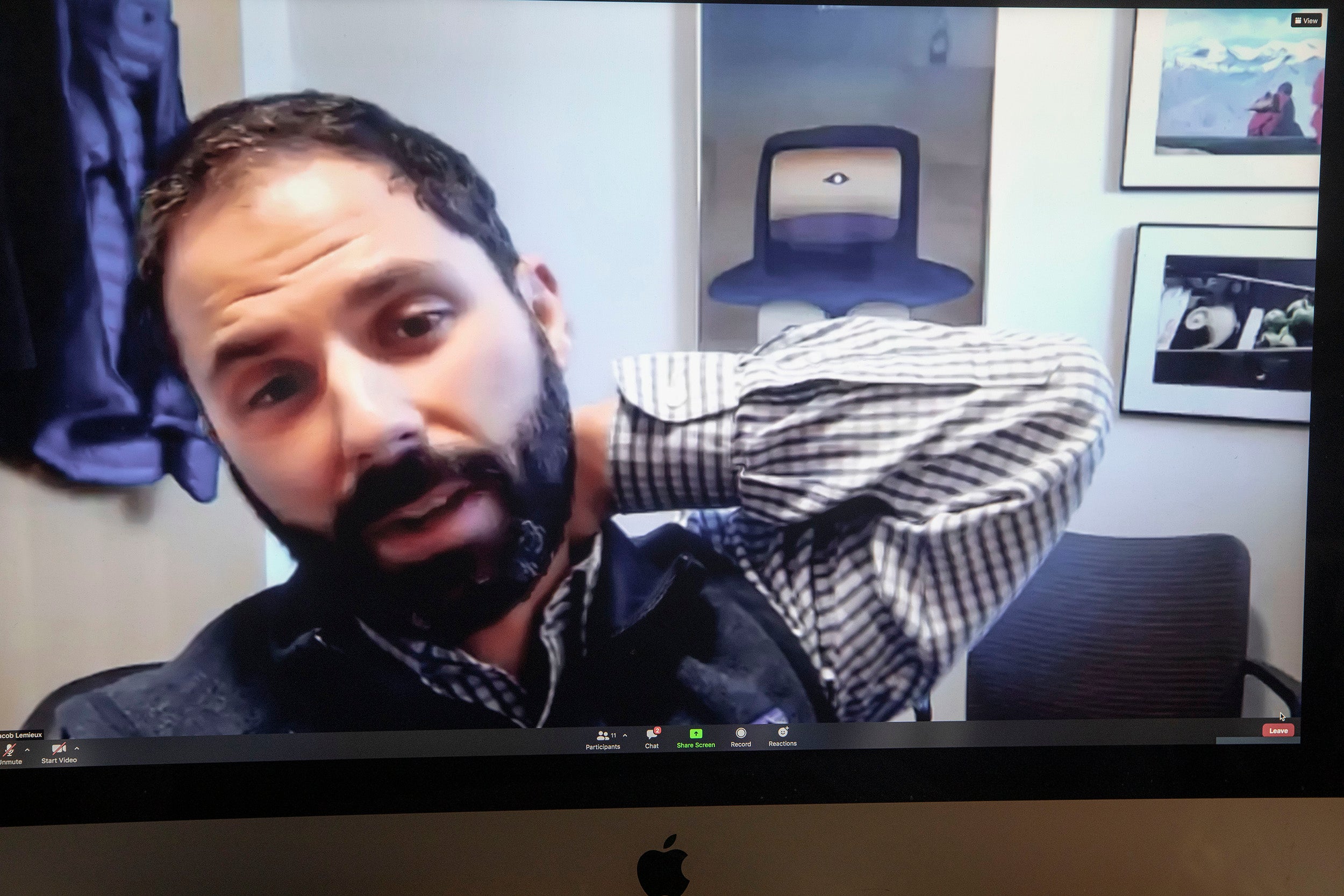
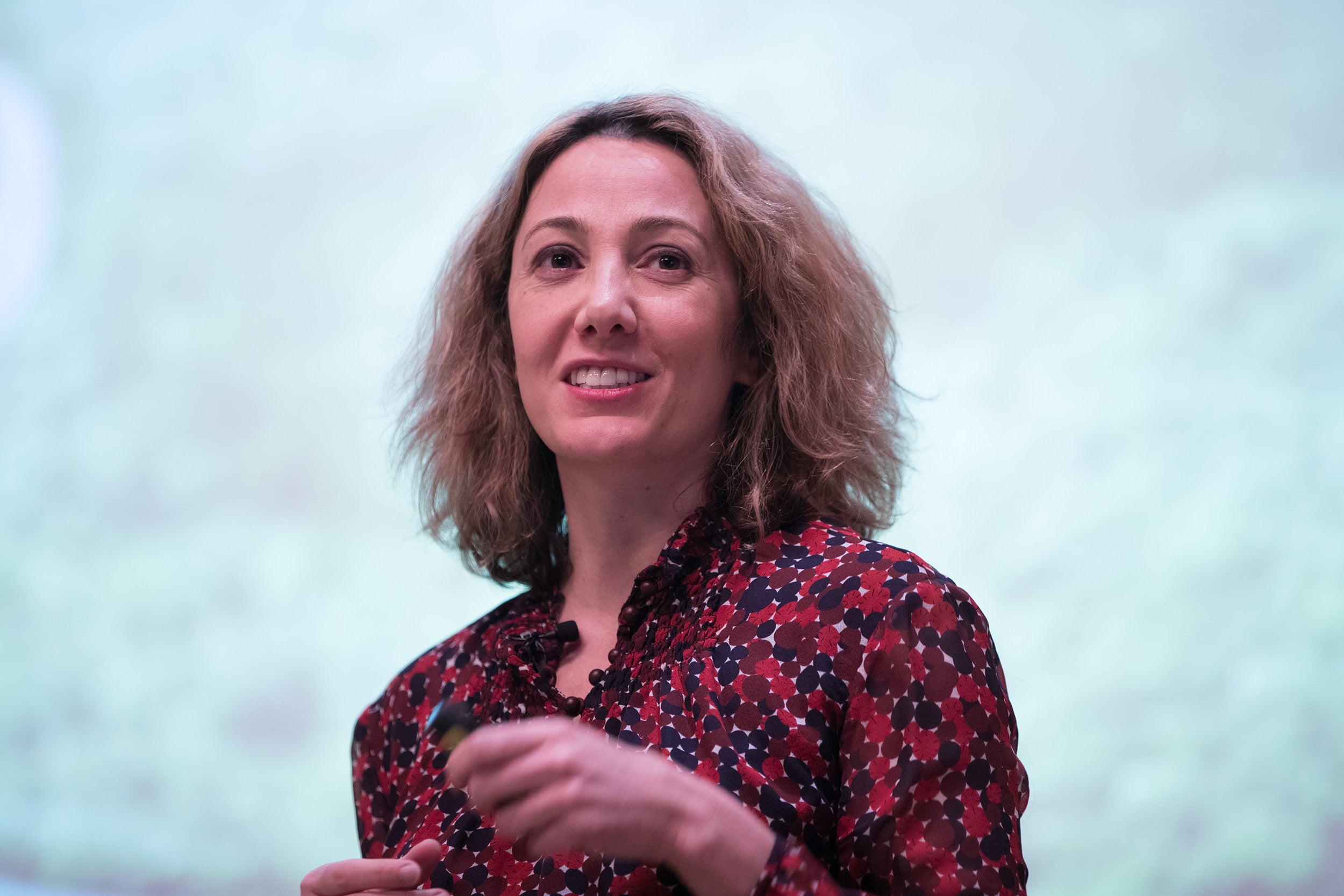
Jacob Lemieux and Pardis Sabeti.
File photos by Kris Snibbe/Harvard Staff Photographer and Dina Ruddick
GAZETTE: I think the number that you analyzed — 6 million genomes — would surprise most readers, if you’re talking about unique genomes. How many are there?
LEMIEUX: What we call a genome is a sequence typically from an individual patient. We tend to think of one genome representing one patient’s virus. That’s a pretty good approximation of what’s in the database. But each patient’s infection corresponds to many millions of copies of the virus, so it’s a tiny fraction of the number of SARS-CoV-2 replication events that have occurred in the pandemic.
GAZETTE: Are there at least small variations in the virus in every patient’s body?
LEMIEUX: There are small variations within a given person, but we don’t need to model them all to understand the pandemic. In fact, many of the viral sequences across different individuals are identical at the consensus level. So there are not 6.5 million unique genome sequences. Some are identical. That’s actually what we track, and we even coarsify [generalize] the data to the level of lineages, which are essentially genetically similar groups of genomes that we consider together. Then we ask, in different populations over time: Do we see more of that group of genomes called “the lineage” or fewer of that group of genomes over time? For the purposes of this model, we use 3,000 lineages and each contains a unique constellation of mutations. The mutations, though, can occur in more than one lineage. And that’s where we’re able to get the power to ask which mutations are responsible for a lineage growing over time or dying out. And, because people all around the world are contributing genomes to these databases, we have essentially a real-time view of which lineages are growing in which places, sometimes due to random chance, like a big super-spreading event. But if we find that the same lineage is dominating in Massachusetts and New York and California, that tells us there’s probably something about that lineage. We’re able to infer what that is by doing the same thing for mutations. If we see a mutation like N501Y, for example, that is consistently found in lineages that tend to grow, then we think that there’s something about that mutation that causes that lineage to grow in a population.
GAZETTE: Can this model predict future variants that might arise, or is it really working with existing genomes, sorting out the thousands of lineages for ones that might spread? Can it actually look ahead and say, “Well, this is likely to mutate here. And that’s going to be a problem”?
LEMIEUX: Sort of both. One thing it does well is provide an estimate of the growth rate of the different lineages that are currently circulating. We assign a fitness to every mutation that’s been observed in the population, and if a mutation has never been observed before, we can’t assign it a fitness. So, if there’s a hypothetical strain from combinations of mutations that have been observed in other places, but not brought together in the same lineage before, we can forecast the growth rate for that strain. If we haven’t observed the mutations, the model doesn’t know the effects from that particular mutation.
GAZETTE: How did the work get started?
SABETI: Jacob, as a then-medical-student-turned-postdoc, and another graduate-student-turned-postdoc, Danny Park, had long been investigating methods to detect adaptive variants in microbes, starting with malaria — it was a passion project of the lab’s. Our early work was in detecting natural selection in humans and other mammals, and the challenge there is that, because the generation times are so long, we have to infer historical events. In infectious diseases, what’s amazing is we get to see natural selection unfold before our eyes. We can track it in real time. That’s the power of this approach.
More like this
But when Jacob and others began this work on malaria a decade ago, the data was just too sparse. Amidst Ebola, we began to get higher-density data and published work with Jeremy Luban [at the University of Massachusetts Chan Medical School] identifying variants that rose in prevalence. But there was still too little data to make statistical inferences of the nature we can now. With the pandemic, we switched very quickly from a situation in which we didn’t have enough data to a situation we had so much data that people weren’t able to manage it. And it was very heterogeneous data: We didn’t know the data sources; we didn’t know the quality of the sequences and so how to curate and basically tame that massive data set to get robust results.
LEMIEUX: At the time, we weren’t used to working with millions of microbial genomes. We were used to dealing with hundreds or thousands. That’s when we started working with the PyR0 team at Broad, who had come from Uber AI, where they had built this probabilistic programming language to do computation on really large data sets. Fritz Obermeyer was the main person working on this project. He was able to put together a model that made sense of what lineages are transmitting more readily and growing more quickly in the population and represented those lineages by their constituent mutations. The other critical innovation from Fritz’s work is that it can run on modern processing hardware, using innovations in software engineering and modern computing power. That made this possible in a way that wouldn’t have been possible before.
GAZETTE: How important was an interdisciplinary approach in this research? It sounds like you had a lot of different folks involved.
SABETI: This is at the interface of what we call “variant-to-function,” and individuals from mathematics, computer science, and computational biology came together with virologists, molecular biologists, infectious disease researchers, and clinicians. By going from bench to bedside, you see patterns and become intrigued by them.
GAZETTE: Clearly the ability to predict variants and which ones are going to dominate is important. What do you see looking ahead with this model?
SABETI: The Holy Grail the field often looks to is the ability to predict from the outset which mutations will be important and what their effects will be, essentially how a microbe will adapt. To do so, we will need these massive models to really interrogate viral and microbial genomes and, when you see different mutations enough times, start figuring out the patterns and underlying logic. I think we can get to the point where we begin to understand how adaptation is going to happen and how we should address it in the development of our countermeasures, but it will require a lot of data. Whenever people ask, “Have we generated too much data?”, I argue that we haven’t by a long shot. We really should get to the point that it becomes routine to sequence every single microbial genome detected in infections because there are things we don’t even know are possible to ask yet because we don’t have the data.